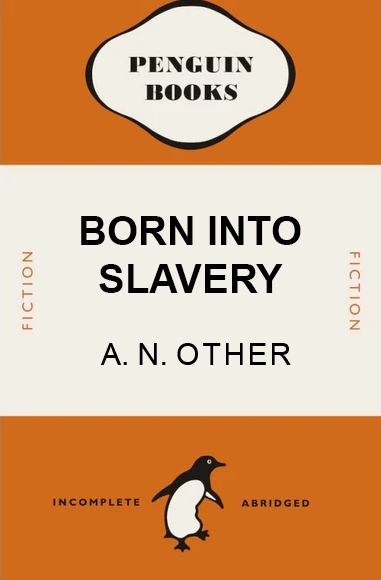
This is a game to see if you can identify the original story author in the generated text below. It has a new title: Born Into Slavery. The generated text is followed by the classic story which was used as the prompt for the computer generation. For technical details see end.
Born Into Slavery
What would occur when the daughter came for a visit? Her daughter could not wish to come here but if she had to she was certain to be disappointed.
When her eldest daughter was in her early thirties, Mr. Smith thought it might be advisable to take her away with him to her own country. The time had been long since passed; what had gone before was now forgotten. She was quite happy at home, though she knew the consequences of her leaving the family.
Mrs. Smith, who lived on her own farm, was the more interested of the two, as she had a son she loved. She would rather she had one of her own daughters than, with him, a son. As it was she was obliged to take him along, though with a slight advantage in health and strength. The child, however, was well behaved, and was very fond of her.
The old couple would have had a child when Mrs. Smith was dead; the latter would not have died till Mr. Smith, as they thought, had been ninety years old, and with her, after him. The child, as they said, would live till old Mr. Smith had died; if that should prove impossible, they would have had an heir to one of the first families to the west of the river.
Mrs. Smith was still an old woman, she remembered; her eyes had become dim, her hands and feet were weak, her feet were dull and bent, her hands wrinkled and loose. A change from her old condition, she thought, would have been too much for her now. She had always been happy in her old condition, though always in the present mode.
This story made her do something she thought necessary for the little girl, and she went into the hall and sat down in the great old chair opposite to Mrs. Smith
A woman’s strength, she thought, was her knowledge of her father’s affairs. She knew that as long as her children could walk with her, they could not be married.
Her husband knew that too; he knew where he was going, what he was going to do, and was never the same afterwards. There was a very large number of children, her daughters, living abroad, in the most irregular manners. He had grown somewhat deaf, with a peculiar difficulty about their voices.
And Mrs. Smith never liked it when she was in any degree unhappy; she could not bear to see her men, much less her children, so miserable. Every time they came she wanted to have a chat with them. She was a woman of very little self-control, and she would have felt an extraordinary strain in talking to men in whom she was so anxious to be connected. She had grown quite aware of the dangers of these visits. She felt that she had the moral to avoid them. It was the same with her husband.
One of the dangers she had to observe was whether they should try to give her good advice on an important subject; then she could say nothing but good things about them. And she did not give you any reason why.
And so he took her into another house, where she found him dead. Here is the explanation of one of our own writers, that was born into slavery, and who has since been made a public writer.
In the first place, we say that his wife knew, but she did not have sufficient excuse for going back to him. For she had been married to his eldest son, whom he kept with her, but she had never had a son, not that she was less prone to be the subject of any kind of cruelty than any other woman.
She was a woman well grounded, and could be trusted not to become a mistress or to marry a knave. Now, the reason why Master Smith was the property of Mr. Smith was, that there were several houses there, called for the use of this one Mr. Smith, and he was not there, but was there at a different time; so he did not know him or know of his own family.
But of course this also became a reason for the destruction of his father’s property; for those other houses were to be used by Mr. Smith at a later time, and hence there was no excuse to lose them. And he knew of the condition of his father’s property, and that he had been made a public writer.
Now he said to Master Smith,
“What is the reason of your going to me at this time, that I do not go to him myself?”
“Well, I have said to him before, I do not go to him, because my father was in debt to you.”
“How did I know it?”
“He had a contract of sale of my house, for you had a lease for the premises. You and your father made a bargain, and did not pay; but he does me to make good my debt. He gave me this lease, but left the property to me.”
“What can I do for it?”
“I will not go with you.”
And Mr. Smith went away in his ship; and while he was doing this, he heard the voice of his father’s son, who was living in the ship, saying to him,
“Your father has made you a public writer, at a time when I should have told you everything that you ought to know about me; and he is coming to me to sell my property for your property, and that we must not meet there till the day after the feast.”
As it happened, at the end of his talk with Mr. Smith, who would then have come to him, he heard of the destruction of Mr. Smith’s house, and of the damage done, for a great many months afterwards, to the houses belonging to his son, because of that man’s writing; and Mr. Smith went away in his ship, that there might be no necessity of his telling Mr. Brown that he might not receive anything from him from him without reason.
And it was, at that time, a great pleasure to him to have the ship and his house in the same box, and he said nothing of it.
Original
Pride and Prejudice
Jane Austen 1813
It is a truth universally acknowledged, that a single man in possession of a good fortune, must be in want of a wife.
However little known the feelings or views of such a man may be on his first entering a neighbourhood, this truth is so well fixed in the minds of the surrounding families, that he is considered the rightful property of some one or other of their daughters.
“My dear Mr. Smith,” said his lady to him one day, “have you heard that Manorfield Park is let at last?”
Mr. Smith replied that he had not.
“But it is,” returned she; “for Mrs. Long has just been here, and she told me all about it.”
Mr. Smith made no answer.
“Do you not want to know who has taken it?” cried his wife impatiently.
“You want to tell me, and I have no objection to hearing it.”
This was invitation enough.
“Why, my dear, you must know, Mrs. Long says that Manorfield is taken by a young man of large fortune from the north of England; that he came down on Monday in a chaise and four to see the place, and was so much delighted with it, that he agreed with Mr. Morris immediately; that he is to take possession before Michaelmas, and some of his servants are to be in the house by the end of next week.”
“What is his name?”
“Bingley.”
“Is he married or single?”
“Oh! Single, my dear, to be sure! A single man of large fortune; four or five thousand a year. What a fine thing for our girls!”
“How so? How can it affect them?”
“My dear Mr. Smith,” replied his wife, “how can you be so tiresome! You must know that I am thinking of his marrying one of them.”
“Is that his design in settling here?”
“Design! Nonsense, how can you talk so! But it is very likely that he may fall in love with one of them, and therefore you must visit him as soon as he comes.”
“I see no occasion for that. You and the girls may go, or you may send them by themselves, which perhaps will be still better, for as you are as handsome as any of them, Mr. Bingley may like you the best of the party.”
“My dear, you flatter me. I certainly have had my share of beauty, but I do not pretend to be anything extraordinary now. When a woman has five grown-up daughters, she ought to give over thinking of her own beauty.”
“In such cases, a woman has not often much beauty to think of.”
“But, my dear, you must indeed go and see Mr. Bingley when he comes into the neighbourhood.”
“It is more than I engage for, I assure you.”
“But consider your daughters. Only think what an establishment it would be for one of them. Sir William and Lady Lucas are determined to go, merely on that account, for in general, you know, they visit no newcomers. Indeed you must go, for it will be impossible for us to visit him if you do not.”
“You are over-scrupulous, surely. I dare say Mr. Bingley will be very glad to see you; and I will send a few lines by you to assure him of my hearty consent to his marrying whichever he chooses of the girls; though I must throw in a good word for my little Lizzy.”
“I desire you will do no such thing. Lizzy is not a bit better than the others; and I am sure she is not half so handsome as Jane, nor half so good-humoured as Lydia. But you are always giving her the preference.”
“They have none of them much to recommend them,” replied he; “they are all silly and ignorant like other girls; but Lizzy has something more of quickness than her sisters.”
“Mr. Smith, how can you abuse your own children in such a way? You take delight in vexing me. You have no compassion for my poor nerves.”
“You mistake me, my dear. I have a high respect for your nerves. They are my old friends. I have heard you mention them with consideration these last twenty years at least.”
“Ah, you do not know what I suffer.”
“But I hope you will get over it, and live to see many young men of four thousand a year come into the neighbourhood.”
“It will be no use to us, if twenty such should come, since you will not visit them.”
“Depend upon it, my dear, that when there are twenty, I will visit them all.”
Mr. Smith was so odd a mixture of quick parts, sarcastic humour, reserve, and caprice, that the experience of three-and-twenty years had been insufficient to make his wife understand his character. Her mind was less difficult to develop. She was a woman of mean understanding, little information, and uncertain temper. When she was discontented, she fancied herself nervous. The business of her life was to get her daughters married; its solace was visiting and news.
Notes
Prompt was 10-20 words from the original. GPT-2 system by Fabrice Bellard – see the site below for credits etc.
Made on my creativity app Story Live – please visit.
Text from Gutenburg free classic ebooks
A certain amount of cherry-picking of the most grammatical and interesting generated parts, but no actual editing (moving around of words or new words). Identifying names are changed.
More of these will be posted, see the index.